Recommendation Engines. How Algorithms Are Affecting Our Decision Making Everyday!
What are Recommendation Engines
In the past, when people had to make choices, like selecting a TV, picking a movie, or deciding on a vacation spot, they typically leaned on a mix of friends’ advice, online reviews, or even expert opinions. With the boom of online shopping, these decisions have grown more challenging. Suddenly, we’ve shifted from a time of limited choices to a vast sea of options — thanks to the internet.
This is where recommendation systems step in, offering a guiding hand in our sea of choices. From suggesting movies and linking friends on social platforms to optimizing search results and even proposing dinner spots, these algorithms subtly steer our choices.
At their heart, these systems are complex algorithms analyzing user data and interactions to serve up relevant suggestions. They aim to refine our online experiences, tailoring them based on our history and inclinations.
E-commerce platforms provide a clear example. Ever notice how after buying or browsing a specific product, you’re presented with similar options? That’s the recommendation system at work, nudging you towards items it believes align with your tastes. For instance, after buying a pair of shoes from a specific brand, you might see other styles from that brand during your next visit.
Examples of Recommendation Engines
Below are some other examples of how recommendation engines are used in various industries:
Consider music platforms like Spotify. They utilize recommendation systems to curate song, artist, and playlist suggestions grounded in a user’s past choices, saved tracks, and followed playlists. Interestingly, they also factor in one’s mood, deciphered from the tracks and playlists one tunes into at different hours.
Similarly, dating apps like Tinder employ these engines, but with a different focus. They recommend potential matches based on preferences, swipes, matches, and details like age, location, and interests.
In the realm of journalism, digital outlets like The New York Times leverage recommendation algorithms. They offer readers articles influenced by their reading habits, saved stories, or even shared pieces. An article’s general traction among readers also plays a role.
For gamers, platforms such as Steam utilize recommendation systems in a unique way. Their suggestions on what games to play next are shaped by one’s previous purchases, played games, individual preferences, and a game’s overall popularity among the community.
Think of travel booking sites like Expedia. They have engines in place that push hotel, flight, or vacation package recommendations our way, all based on our past bookings and searches. These systems also weigh our personal preferences and see where everyone else is headed to offer the best suggestions.
Then there’s LinkedIn, our professional social media hub. It suggests connections not just based on who we’ve connected with before, but also where we’ve worked and our skill set. Ever noticed how it also recommends companies or profiles that seem popular among your contacts? That’s the algorithm at play.
For the foodies, apps like Grubhub suggest restaurants and dishes, considering what we’ve ordered before or even marked as a favorite. It smartly blends in factors like our current location and the dining spots or dishes that are trending in the community.
Lastly, if you’re on Coursera or similar online learning platforms, the courses or programs you see recommended aren’t random. They’re aligned with what you’ve previously enrolled in or completed. Moreover, if a course is getting rave reviews and seeing high enrollment, you’re more likely to spot it in your recommendations.
Recommendation systems have woven their way into various industries, aiming to offer tailored suggestions to users by analyzing their past actions and likes. These systems aren’t just about helping users find new items or content; they’re also about boosting user engagement and potentially amplifying a business’s sales.
A Glimpse into Amazon’s Recommendation Strategy
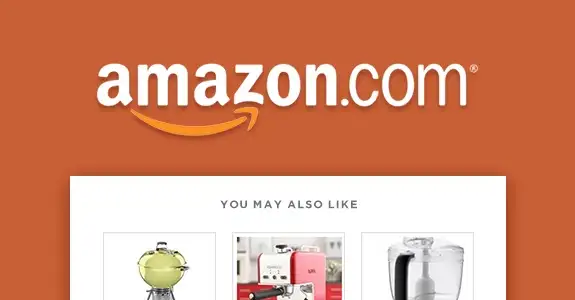
Amazon masterfully employs recommendation systems to tailor product suggestions for its shoppers. What a user sees recommended isn’t just arbitrary; it’s a blend of their purchase history, products they’ve browsed, and items they’ve rated or left feedback on.
At its core, Amazon’s recommendation approach seeks out patterns. Say, for instance, a shopper often buys novels from a certain writer. The system might then spotlight other books from that author or even delve into similar genres. But it’s not just about individual patterns; the reviews and ratings, plus how well a product is doing in its category, all come into play.
Moreover, there’s a community aspect. If you’re eyeing a specific product, Amazon might nudge you with suggestions that have been a hit among other users who looked at or bought the same item.
Ultimately, through these intelligent, tailored product suggestions, Amazon aims to keep users engaged, possibly leading to increased sales for the e-commerce giant.
How Dating Sites Use Recommendation Engines
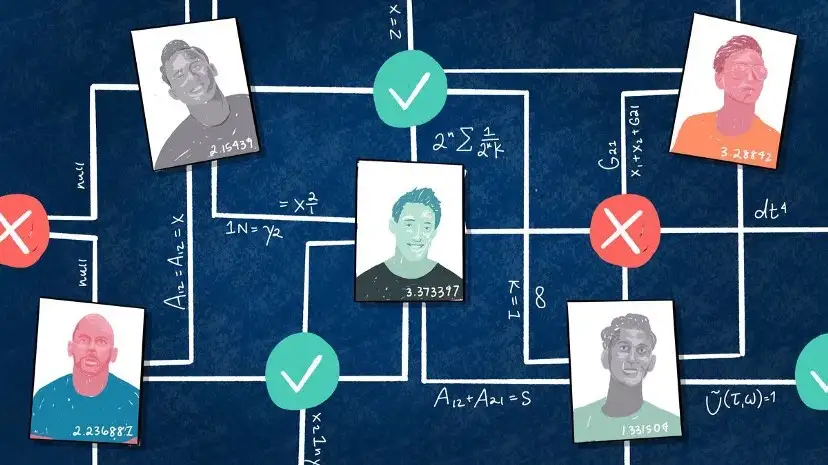
Dating platforms like Tinder are pretty sophisticated beneath the surface. It’s not just a random assortment of profiles you’re swiping on; it’s the result of intricate recommendation systems working behind the scenes. These systems dive deep into user preferences, past interactions on the app, and even factors like age, location, and specific interests to present the most likely matches.
Imagine a user who’s shown a preference for profiles around their age and residing in the same city. The system doesn’t merely bank on that information. It merges these preferences with past interactions — the swipes, the matches — and even weighs how popular a profile is among all users.
But it doesn’t stop there. Elements like shared acquaintances or overlapping interests can be the cherry on top. By weaving in these details, the recommendation system ensures users might stumble upon matches they’d otherwise have overlooked, adding a layer of serendipity to the science of matchmaking
The Science Behind Social Media Suggestions
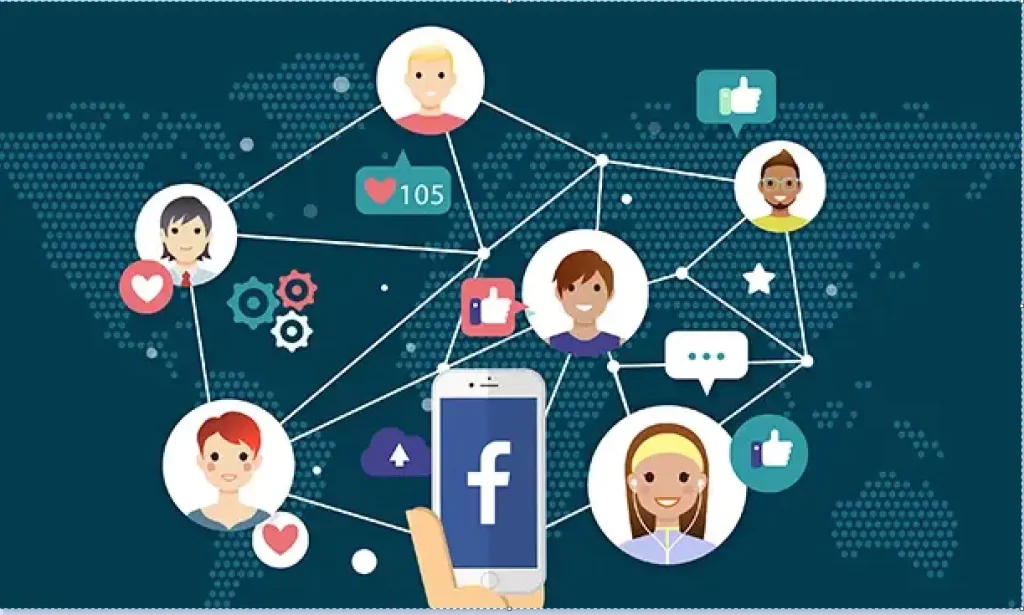
Ever wondered why LinkedIn or Facebook suddenly suggests that colleague from a past job or a classmate you hadn’t thought of in years? It’s not sheer coincidence; it’s all about the power of recommendation engines.
Dive into your LinkedIn account. The platform intricately studies your professional journey — from job titles, industry sectors, to geographical locations. Combine this with a sprinkle of your previous connections and interactions, and voila, a tailor-made list of suggested connections pops up. So, if your network is bursting with professionals from, say, the tech world, LinkedIn’s engine will likely nudge you towards similar industry profiles.
On Facebook, it’s a similar story but with a more personal twist. Apart from your existing friend circle, the platform examines other aspects like the pages you’ve visited or groups you’re a part of. The goal? Craft suggestions that don’t just expand your network but resonate with your interests and past interactions.
In essence, these platforms leverage intricate algorithms to make our social media experience feel tailored, almost intuitive, creating a seamless blend of science and social connection
Types of Recommendation Engines
There are several different types of recommendation systems, each with its own unique characteristics and capabilities.
- Content-Centric Recommendations
Content-based recommendation systems make their picks based on attributes inherent to items. Think of a streaming service that suggests a movie — it’s probably because the film shares genres or actors with others you’ve seen. It’s like having a friend who only suggests books because they know the kind of authors you like. - The Power of Collective Preference:
Collaborative Filtering Ever been to a book club? Collaborative filtering is somewhat like getting book suggestions from club members. This system looks at user behaviors, pooling together likes or dislikes. If people with tastes similar to yours loved a particular book or movie, chances are, you’ll get it as a recommendation. - Best of Both Worlds:
Hybrid Systems Imagine combining the best attributes of both systems mentioned above; that’s a hybrid system for you. By studying item attributes and collective user behaviors, these systems pack a powerful punch. They’re typically more nuanced and often yield more accurate suggestions. - Demographics-Driven Recommendations
Finally, we have demographic-centric systems. These make recommendations based on personal attributes like age or location. For instance, if you’ve ever wondered why certain genres pop up on your music app, your age bracket might have something to do with it. It’s a bit like a billboard targeting — a general suggestion for those passing by, not super personalized but effective for a broad audience.
Unpeeling the Banana Paradox in Recommendation Systems
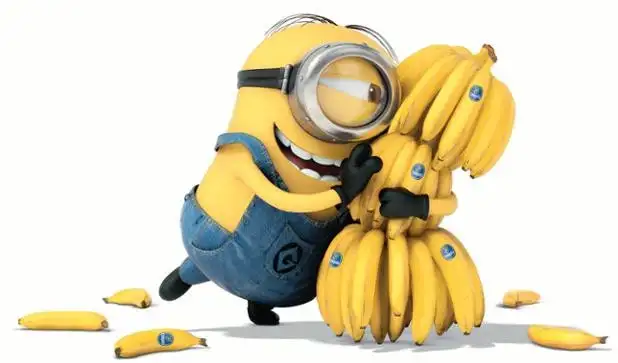
The “Banana Paradox” aptly captures a pitfall in the realm of recommendation engines. It’s when the system, ensnared by a user’s past behaviors, keeps suggesting strikingly similar items, resulting in monotonous, undiverse recommendations.
So, why the fruity name? The term was coined due to a quirk in grocery recommendation systems. Since bananas are a ubiquitous buy — almost everyone adds them to their cart — everything in the store was being associated with bananas. The result? A bizarrely strong recommendation link between bananas and, well, just about everything else.
Consider an EDM enthusiast with a playlist saturated with those beats. If the system keeps pushing more EDM, it’s as if they’re trapped in a never-ending electronic dance party. They might crave jazz or blues but are incessantly handed more synths and drops. Such limited exposure can disengage users and diminish the value they see in the recommendation engine.
Tackling the Banana Paradox requires a more holistic approach. Systems might need to:
- Gauge the user’s present mood or context.
- Leverage collaborative filtering, factoring in what diverse user groups enjoy.
- Blend content-based and collaborative filtering strategies, for a more rounded recommendation.
Ultimately, by introducing diversity and considering varied dynamics, recommendation engines can serve up more engaging, unpredictable, and appreciated suggestions.
The Cold-Start Problem
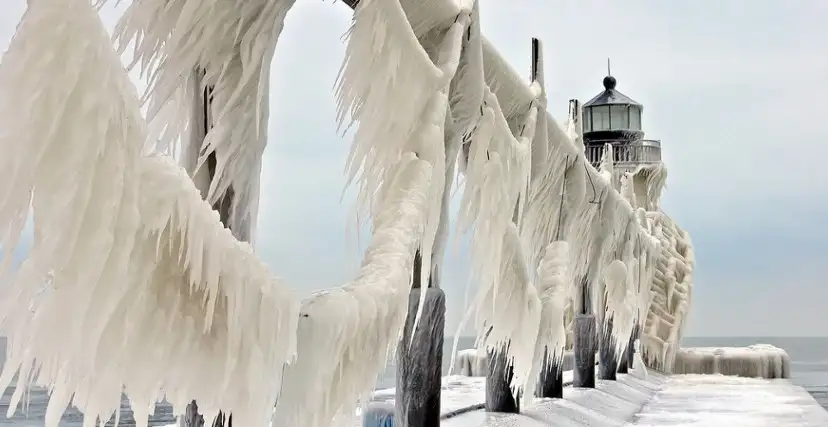
In the realm of recommendation systems, the “cold start” problem emerges as a prominent challenge. At its core, this issue is about a system’s struggle to offer relevant suggestions when encountering new users or items devoid of any historical data. Traditional recommendation engines rely heavily on past interactions and behaviors to fine-tune their suggestions. Without this historical backdrop, generating meaningful recommendations becomes a herculean task.
Tackling the Cold Start Problem: A Multifaceted Approach
Data Collection Initiatives:
- Why: The foundation of any recommendation system is data. In the absence of historical interactions, actively gathering data can offer an initial understanding.
- How: Deploy surveys or interactive quizzes during user onboarding. Ask users to set up profiles, highlighting their preferences or past experiences. This acts as a starter kit for tailoring early recommendations.
Lean on Default Recommendations:
- Why: While these may not be hyper-personalized, they serve as a starting point.
- How: Promote items or content that are universally popular or trending. Alternatively, suggest items that have attributes commonly liked by the broader audience.
Side Information as a Savior:
- Why: Even without direct interaction data, side information can provide valuable context.
- How: If you know a user’s demographic details, such as age or location, this can be a goldmine. For instance, a music streaming service might curate region-specific playlists for a user based on their location.
Collaborative Filtering Techniques:
- Why: By examining patterns in similar users, it’s possible to bridge the data gap for newcomers.
- How: If a group of users shows similar behavior, and a new user displays a pattern resembling that group, recommendations can be made based on the group’s overall preferences.
The Power of Hybrid Systems:
- Why: These systems integrate the strengths of both content-based and collaborative filtering, offering a comprehensive solution.
- How: Such a system might first use content attributes to offer initial suggestions. As the user begins to interact, the system pivots, integrating collaborative data from similar users to refine further and personalize the recommendations.
Revolutionizing Hotel Sales with Recommendation Systems
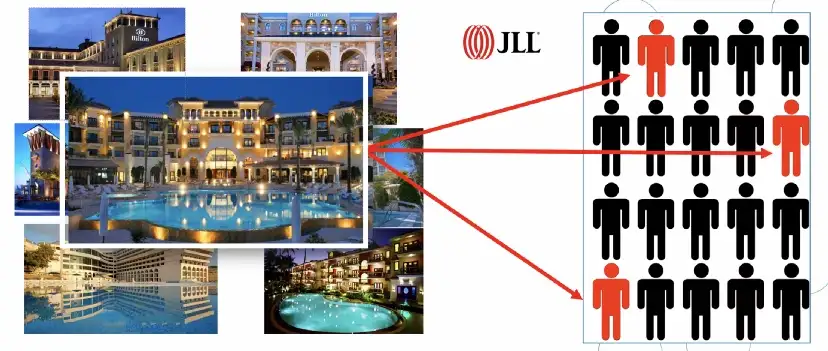
Harnessing the power of recommendation engines can significantly optimize the sales pipeline in the hotel industry. These sophisticated systems use historical data, coupled with intricate algorithms, to connect potential buyers with suitable hotel properties. The results? Faster, more streamlined sales and an enhanced user experience.
Advantages of Integrating a Recommendation System in Hotel Sales:
- Efficiency Boost: Traditional property sales, often spanning months, can be trimmed down to mere seconds. This swift matching process can lead to substantial cost savings, with potential annual savings soaring over $10 million.
- Automation Advantages: By automating the buyer-property matching procedure, there’s a significant reduction in manual labor. This not only minimizes human error but also frees up brokers, allowing them to concentrate on more strategic responsibilities.
- Data-Driven Insights: An analytics-rich platform, powered by the recommendation engine, can offer real-time insights, enabling more informed decision-making throughout the sales process.
Case Study: Analytica Data Science Solutions and Jones Lang LaSalle
In 2016, Analytica Data Science Solutions collaborated with real estate giant, Jones Lang LaSalle, pioneering a state-of-the-art recommendation engine. With a robust database featuring tens of thousands of hotels and over 200,000 potential investors, the primary goals were clear:
- Accelerate sales timelines.
- Transform manual, time-consuming processes into automated, efficient ones.
- Seamlessly match a plethora of hotel properties with the right investors.
Empowered with vast datasets, including historical hotel sales data and potential investor profiles, the data scientists architected a revolutionary recommendation system. This engine could instantaneously match properties with potential buyers — a process that formerly took three months. The game-changing system can be accessed here.
In Essence…
Recommendation engines, with their ability to tap into past behaviors and preferences, are reshaping the digital landscape. E-commerce giants, music streaming services, and even social networking sites employ these tools to curate personalized experiences, heightening user engagement. With varied models available, like content-based, collaborative filtering, hybrid, and demographic systems, these engines are versatile, catering to diverse needs. Their potential is not limited to just enhancing user experience but extends to tackling challenges like the cold start problem, marking them as pivotal tools for myriad platforms.
Read More blogs in AnalyticaDSS Blogs here : BLOGS
Read More blogs in Medium : Medium Blogs